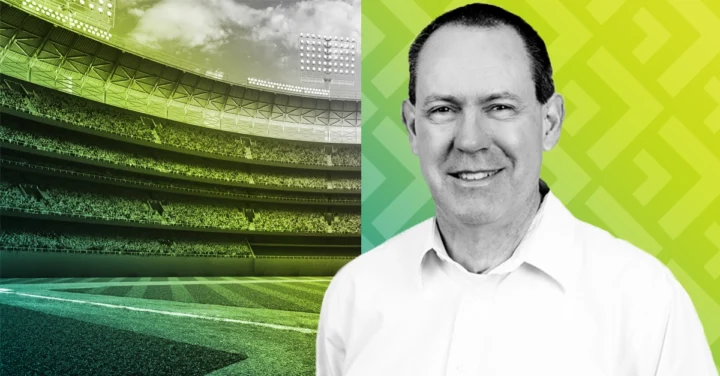
By Chris Fievoli, FCIA
At the risk of this being an annual event, I am once again trying to make a connection between actuarial science and baseball. The inspiration for this piece comes from June 28, 2023, when Yankees pitcher Domingo Germán pitched a perfect game against the Oakland Athletics. Twenty-seven batters faced, twenty-seven batters retired. Nobody reached first base.
A perfect game is one of the sport’s rarest accomplishments, having been achieved only 22 times since the modern era of baseball started in 1903. One would think that it reflected superior pitching talent, especially when you consider some of the players that have done so – Cy Young, Sandy Koufax, Catfish Hunter, Randy Johnson and Roy Halladay. If that was your starting five, your team would probably win 130 games.
Historical examples of perfect games
However, some lesser lights have also thrown perfect games. Dallas Braden did so in 2010 but would win only 25 other big league games. Even so, that’s a better record than Philip Humber, who threw his gem in 2012, and finished with a career record of merely 16-23.
The most noteworthy example might be Don Larsen, who stunned the baseball world on October 8, 1956, when he set the Brooklyn Dodgers down in order in Game 5 of the World Series. No one expected such a performance from a pitcher who had gone 3-21 two years previously. Larsen would pitch eleven more years, and finish with a decidedly average 81-91 won-lost record. Famous forever, but nowhere near Hall of Fame worthy.
Perfect games: skill or fluke?
So, which is it? Does a perfect game represent pitching supremacy, or is it just a fluke? To dig a bit further, perhaps we can try to estimate the probability of throwing one of these gems. If, all other things being equal, the probability of retiring a batter is p, then the probability of a perfect game is p27.
To illustrate, let’s go back to Larsen, and compare him to another player that was on the Brooklyn roster that day – the aforementioned Sandy Koufax. (And, we’ll only use his stats from 1961 to 1966, when he magically transformed from an average hurler to the best pitcher on the planet.)
Thanks to the folks at Baseball Reference, we can see that Koufax faced 6,463 batters in that time period, and gave up 1,171 hits and 412 walks, for a p value of 0.755. (Baseball fans will recognize that number for something else.[1]) Larsen, over his career, faced 6,708 batters, and surrendered 1,442 hits and 725 walks, for a p of 0.677. If we plug these values into our formula, we see that Koufax had a 0.051% chance of throwing a perfect game, while Larsen’s value came in at 0.003%, which implies that Koufax was 17 times more likely to accomplish the feat, a ratio that probably makes intuitive sense to fans of the game.
The role of randomness in perfect games
However, let’s try looking at this a different way. Suppose that, in an unrelated conversation, I asked someone to assess an event that has a 0.051% chance of occurring. They’d likely say that the probability was so small that it was effectively zero. If I had the same conversation with someone else, and instead used the 0.003% figure, they would probably come up with the same answer – mark it zero.[2]
This is the dichotomy we are faced with when dealing with such small probabilities. One event is mathematically 17 times more likely, but at the same time, is practically the same. And there is no way that I could ever pretend that my simple model to estimate the chance of throwing a perfect game is precise to five decimal places. This is clearly a case of spurious accuracy.
What’s happened is that we have crossed over into a world where randomness trumps everything else. It would certainly be correct to say that perfect games are undoubtedly random events. Dallas Braden’s achievement came a mere 20 days before Roy Halladay’s. However, while these two events were only weeks apart, it wouldn’t be inaccurate to say it all came down to chance, with no perfect games being thrown during the entire decade of the 1970s.
Considering extreme events in actuarial science
Now, what does this mean for actuaries? I think it can be instructive in terms of how we look at extreme events that have a very low probability. If you do any stochastic testing, you know that there are some outcomes living high up in the 99th percentile. And those are the really bad ones – potentially extinction level events for your enterprise.
We tend not to spend too much time thinking about those events, for what may or may not be a good reason. If your attitude is, “They have such a low probability of happening, I don’t need to consider them,” then that may be a problem, for the reasons discussed above. You may be implicitly assuming that the laws of randomness don’t apply, which of course is never true. And remember – once an event happens, the fact that it had a low probability of occurring beforehand becomes immaterial. What was once probabilistic has become binary – either it happened, or it didn’t.
Conversely, I’m not proposing that these outcomes warrant a lot of attention. We certainly couldn’t include a risk margin that covers 100% of possible outcomes – that would be prohibitively expensive. But it may make sense to understand what these extreme events look like, how bad they can be and what are the conditions that could lead to them. This would be better than pretending they didn’t exist.
Striking a balance: acknowledging and mitigating extreme risks
There is a scene in the 1992 film Glengarry Glen Ross when desperate salesman Sheldon Levene (Jack Lemmon) is trying to convince office manager John Williamson (Kevin Spacey) to give him some of the premium client leads that just arrived in the office. Levene repeatedly promises that he will close the deals, but every time he is met with the same response from Williamson – “But what if you don’t?”
“As actuaries, maybe we should be asking a similar question – what if the worst-case scenario does happen? We may not like the answer, but at least the users of our services will understand the risk.”
To quote another Jack Lemmon film – the final line of the movie Some Like It Hot was “nobody’s perfect.” Domingo Germán was perfect for an evening, but there’s no way to keep the world perfectly safe from risk, no matter how good an actuary you are. And that’s OK.
[1] In case you didn’t catch that, Hank Aaron finished his career with 755 home runs.
[2] Gratuitous Big Lebowski reference.
This piece also got me thinking about the role of coincidence, in professional sports and elsewhere. Events which seem overwhelmingly unlikely can and do happen. If we stick to the narrow topic of families professional sports, no one could ever have predicted that:
– A father and son would hit back-to-back home runs in a major league baseball game.
– Twin brothers would win consecutive Art Ross trophies as the NHL’s leading scorer.
– The coaches of the two teams facing each other in the Super Bowl would be brothers.
But these things have all happened in my lifetime. (Bonus if you can identify them without looking it up.)
What do we make of this? I’m not sure, except to say that extremely unlikely events may happen more than we suspect.
Very interesting article. Liked the baseball analogies.