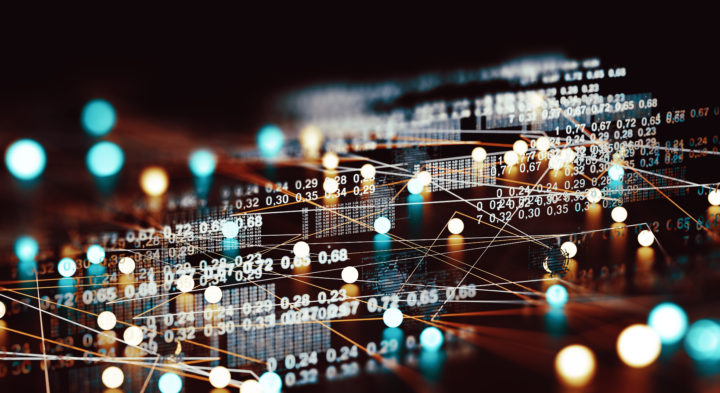
By Joel Li, FCIA
Chair, Committee on Predictive Modelling
Data science has seen tremendous growth as a discipline in the past decade as the amount of information captured about our lives, along with computing power, has grown exponentially.
Data science applications and use cases bring value to every industry imaginable and without a doubt have affected the core industries and practice areas of actuaries. As a result of this quick evolution, data science is not a well-defined term. Nonetheless, it does have working definitions, like the one shared on the IFoA website:
‘Data science’ describes a broad, multidisciplinary field that uses scientific methods, processes, algorithms and systems to extract knowledge and insights from structured and unstructured data. It employs techniques drawn from many fields within the context of mathematics, statistics, computer science, and information science.
In comparison (found on CIA website):
Actuarial science is the discipline that applies specialized knowledge in mathematics, statistical and risk theory to solve problems in insurance, finance, health and other industries.
Data science and actuarial science are both multidisciplinary in nature; they extract insights from data and require strong understanding of the underlying business processes and domain knowledge to be successful at accomplishing the task. What sets data science apart is the novel ways of solving business problems enabled through algorithms previously limited by computation strength and the capability to tap into insights from unstructured data. Or in other words: Data scientists have access to more fancy tools and gadgets to dig into new types of data than what actuarial science offers when it comes to solving the same business problem.
These novel approaches and methodologies have broader applications, hence allowing data scientists to enter more industries and practice areas than the actuaries. A deeper understanding of data science can allow actuaries to leverage data science results in their work and find more applications in emerging practice areas.
Overlaps and application
Big data and predictive analytics are important elements used in the work of a data scientist.
Big data is often first characterized by volume, velocity, and variety.
- Volume – the size and quantity of the datasets. While there is no agreed size threshold for what counts as big data, it is defined as datasets that are too large to be processed by traditional databases or data warehouse solutions.
- Velocity – the speed at which big data accumulates. Big data typically is generated from millions of entities every second of the day. Typical examples of this are telematics data from vehicle sensors, health monitoring wristbands, or browsing history.
- Variety – the heterogeneous source and nature of big data being structured, semi-structured, and unstructured.
While having these characteristics is great and presents new opportunities, it is also important to consider the challenges of using big data brought forward by veracity and variability. Big data can be difficult to match, clean and integrate with the other available data sources. It is also very dynamic and can change frequently. Hence, when working with big data it is critical to consider the tradeoff between the business value of the information versus the complexity and cost of working with big data by focusing on the underlying problem we are trying to solve.
Predictive analytics is the process of drawing insights from historical data to forecast a future event. Often techniques rooted in statistics are used to approximate relationships between a target outcome variable to a range of explanatory variables. This allows data scientists and actuaries to apply these discovered relationships to new datasets to make predictions and forecasts.
The actuarial profession, having been around for well over 100 years, is very familiar with applying predictive analytics, especially to solve the “big data” problems of the past. Actuaries were tackling thousands to millions of individual incidents of mortality, health, or accident claims to draw insights on individual associated risks. This data would have been very difficult to process and accumulate with the limited computing capabilities at the time, hence the “big data” of the past.
Nonetheless, actuaries were able to overcome those challenges through innovation just as how computing advances enable us to solve big data challenges today.
Diverging in approach
While actuaries have always used predictive analytics to build rating plans, or approximation of hazard rate curves for mortality, they tend to look for explainability in models and opt for close-form solutions. Data scientists, on the other hand, prefers algorithmic approaches like gradient boosted models or neural network models due to their better performance as their work tends to focus on the accuracy of the predictions.
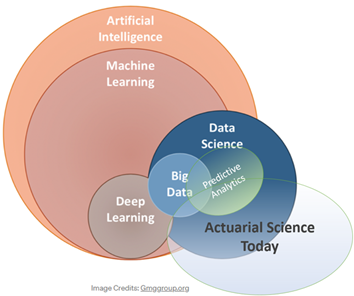
These examples, along with the Venn diagram help to illustrate the prominence of big data and predictive analytics in actuarial work. With technology development on the rise, there are more opportunities for actuaries to sink their teeth into big data and enhance their work. By applying predictive analytics to telematics data themselves, or collaborating with data scientists, there is a lot more work that actuaries can do to improve the prediction and quantification of risks.
Today, there are many data scientists who invest a lot of effort researching how to streamline the explanation of complex model results to help identify its limitations when faced with certain data anomalies. This helps overcome one of the short falls of using algorithmic approaches, and make them more accessible to actuaries. Ultimately, we should always consider the fit for use and feasibility of implementation when applying models or leveraging the work of others.
Artificial intelligence (AI), machine learning, and deep learning?
AI, machine learning, and deep learning are common terms we hear today and are at times used interchangeably. Understanding these terms could help actuaries better communicate with data scientists.
All three could be considered as a field of study; each one more niche than the previous:
- AI is a broad and encompassing term. It could be defined as the theory and development of (computer) systems able to perform tasks that normally require human intelligence. It includes everything from visual and speech recognition to decision-making, translation, and automation of human tasks.
- Machine learning is defined as the use and development of computer systems that are able to learn and adapt without following explicit instructions, by using algorithms and statistical models to analyze and draw inferences from patterns in data.
- Deep learning is a niche area of machine learning that uses large neural network with multiple hidden layers inspired by structure and function of the human brain. These algorithms specialize in tasks such as image recognition and natural language processing.
These fields of study have very broad applications that go beyond data and actuarial science. With the breakthroughs of quantum computing, complex simulations, and algorithms previously limited by traditional computing, operations could soon become possible. Actuaries could be applying these newer techniques to risk modelling and quantification problems such as economic capital modelling, dynamic stress testing, and portfolio optimization.
The bottom line is that while seeming new and somewhat unknown, data science isn’t as different as we’ve come to perceive it. Data and technology are ever evolving and as lifelong learners, actuaries should always be on the lookout for innovative techniques that enhance our work and challenge tradition.